The Power of Data-Centric Artificial Intelligence in 2022 – Analytics Insight
Data-centric artificial intelligence consists of systematically changing/upgrading the datasets to work on the accuracy of your artificial intelligence system
Data-centric artificial intelligence consists of systematically changing/upgrading the datasets to work on the accuracy of your artificial intelligence system. This is generally neglected and data assortment …….
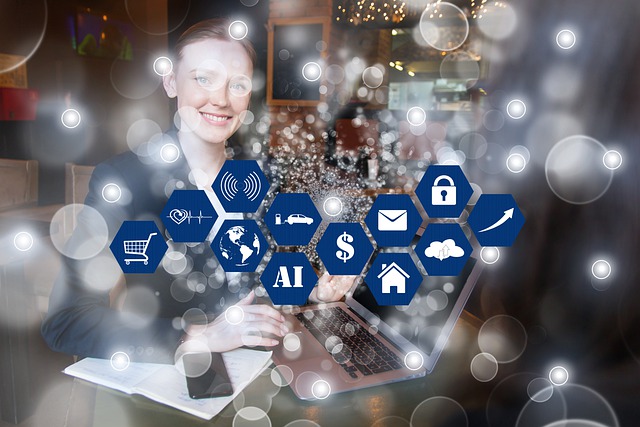
Data-centric artificial intelligence consists of systematically changing/upgrading the datasets to work on the accuracy of your artificial intelligence system
Data-centric artificial intelligence consists of systematically changing/upgrading the datasets to work on the accuracy of your artificial intelligence system. This is generally neglected and data assortment is treated as an oddball task. Most machine learning engineers observe this methodology as really thrilling and promising, one explanation is that it empowers them to try their machine learning models into practice. In contrast, working on data is now and again considered as a low expertise task and many designers like to deal with models all things being equal. Be that as it may, is this accentuation on models legitimized? For what reason does it exist?
The entire range of exploring different avenues with different machine learning models and checking what works best for the given data and business case does not keep the ball rolling for long. Assuming your best data-centric artificial intelligence model doesn’t meet the metric that the business needs to have the option to try out ahead for the venture, comprehend that the time has come to go nearer to the data and burrow further with regards to which piece of the data isn’t qualified to the point of coming to the preparation set. Here you examine assuming there are some particular credits of the test data where your forecasts are a long way from the real world.
Data Label Quality: It could be not too difficult to imagine that the diverse labelers gave various names to an alternate part of data. Assuming there is irregularity as far as how the human specialists see a specific issue, there is a remote possibility that machines will pick it by the same token.
Data Augmentation: Generate the data that your model has not seen during the preparation time. Adding data isn’t the main arrangement consistently. Eliminating the boisterous perceptions prompting the high fluctuation additionally assists the model’s capacity with summing up better on the concealed data.
Data Sources: Many data researchers battle a great deal with this one specifically, as the field includes numerous data sources that go through thorough data understanding, conversations with Subject Matter Experts (SME), tracking down the right business rationale to consolidate them and make one level construction for preparing the machine learning models. There are myriad ways things can go wrong here in terms of schemas, data accumulation, the business logic to make the data coherent, and data storage.
Feature Engineering: Data quality includes improving both – the input data just as the objective/marks. Highlight designing assumes a basic part as far as presenting highlights that probably won’t exist in crude structure, however, the arranged elements can add to significant improvement.
Share This Article
Do the sharing thingy
About Author
More info about author
Source: https://www.analyticsinsight.net/the-power-of-data-centric-artificial-intelligence-in-2022/