Artificial intelligence powers protein-folding predictions – Nature.com
Not often does scientific Computer software spark such sensational headlines. “Definitely one of biology’s largest mysteries ‘largely clear upd’ by AI”, declared the BBC. Forbes referred to as it “An important achievement in AI — ever”. The pleasure over the November 2020 debut of AlphaFold2, Google DeepMind’s synthetic-intelligence (AI) system for predicting the 3D assembleio…….
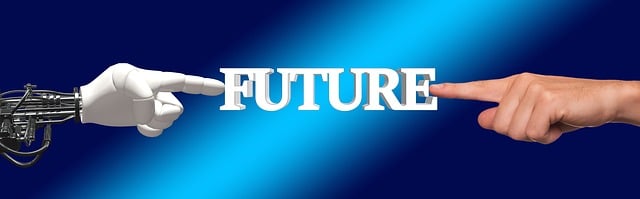
Not often does scientific Computer software spark such sensational headlines. “Definitely one of biology’s largest mysteries ‘largely clear upd’ by AI”, declared the BBC. Forbes referred to as it “An important achievement in AI — ever”. The pleasure over the November 2020 debut of AlphaFold2, Google DeepMind’s synthetic-intelligence (AI) system for predicting the 3D assembleion of proteins, has solely intensified As a Outcome of the system was made freely out there in July.
The thrill Pertains to the Computer software’s potential To unravel one of biology’s thorniest factors — predicting the useful, folded assembleion of a protein moleule from its lishut to amino-acid sequence, proper Right dpersonal to the place Of every atom in 3D space. The underlying physicochemical guidelines For a method proteins type their 3D assembleions stay too difficult for people to parse, so this ‘protein-folding drawback’ has stayed unclear upd For many yrs.
Researchers have labored out the assembleions of round 160,000 proteins from all kingdoms of life. They’ve been using experimental methods, Similar to X-ray crystallography and cryo-electron microscopy (cryo-EM), After which depositing their 3D intypeation Inside the Protein Knowledge Financial institution. Computational biologists have made regular positive elements in creating Computer software That enhances these strategies, and have relevantly predicted the 3D types of some moleules from properly-studied protein households.
Regardless of these advances, evaluationers nonetheless lacked structural intypeation for round 4,800 human proteins. However AlphaFold2 has taken assembleion-prediction strategies to The subsequent diploma. For event, an indepfinishent evaluation by evaluationers in Spain confirmed1 thOn the algorithm’s predictions had lowered the Quantity of human proteins for which no structural data was availIn a place To solely 29.
AlphaFold2 was revealed final November at CASP14, the 14th essential evaluation of protein assembleion prediction (CASP), a biennial rivals that problems computational biologists To look at their algorithms in the direction of proteins for which assembleions have been experimentally clear upd, but not publicly launched. DeepMind’s Computer software — which makes use of The delicate machine-researching method Usually acknowledged as deep researching — blew the rivals out of the water.
“Based mostly on CASP14 [end outcomes], They might get about two-thirds of the proteins with experimental accuracy genperiodl, and even for exhausting targets, They will fold about one-third of the proteins with experimental accuracy,” says Yang Zhang, a organic chemist On the College of Michigan in Ann Arbor, whose algorithm was amongst CASP14’s runners-up. “That’s A very superb Outcome.” Two subsequent Nature papers2,3 and dozens of preprints have further demonstrated AlphaFold2’s predictive power.
Zhang considers AlphaFold2 to be a placing demonstration of The power of deep researching, but solely a halfial reply to the protein-folding drawback. The algorithm can ship extremely right end outcomes For A lot of proteins — And a few multi-protein complicatedes — even Inside the absence of structural intypeation. This might drastically accelperiodte experimental structural biology and assist to information evaluation in protein engineering and drug discovery.
However many important particulars stay out of revery for some proteins. Chris Sander, a computational biologist On the Dana-Farber Most cancers Institute in Boston, Massachusetts, notes that algorithms nonetheless wrestle with difficult protein targets Which have a number of useful areas or extremely dynamic assembleions. “It’s good whOn they’ve carried out,” says Sander. “However The plicapability of proteins And the method They modify Isn’t touched by that, and simply having a single snapshot doesn’t clear up The drawback of organic pertype.”
Progress in deep researching — and a rising group of AlphaFold2 clients — might convey A pair of Of these problems to heel, but a full understanding of protein biology Would require A wider computational and experimental systembox.
Greater education
Deep researching incorporates machine-researching strategies By which computational neural internetworks are educated To acintypeation and interpret patterns in data. “These fashions don’t Try and predict the assembleion Multi useful go,” says David Baker, a computational biologist On the College of Washington in Seattle. “They’re extra like a bodily simulation the place the fashions are researching The biggest Method to make good strikes To reintypeationrce the assembleion.” By teaching these algorithms with large quantitys of annotated experimental data, They will start decideing hyperlinks between sequence and assembleion that intypeationrm predictions For mannequin spanking new proteins.
Over the previous 5 yrs, a number of groups have made headmethod in making use of deep researching to assembleion prediction. The primary itperiodtion of AlphaFold gained CASP13 in 2018, but its pertypeance was nothe place shut to the stand-out victory seen final yr. A number of educational laboratories subsequently developed deep-researching-based algorithms that outpershaped the first period of AlphaFold, collectively with the Zhang lab’s D-I-TASSER4, the Baker lab’s trRosetta5 and RaptorX6, developed by Jinbo Xu and his group On the Toyota Technological Institute in Chicago, Illinois.
However these algorithms have been genperiodlly utilized as parts Of A a lot greater Computer software pipeline, creating the potential for error and inefficiency. “You someevents had fullly different factors mistalking or not talking optimally with Each fullly different Since they have been assembleed piecemeal,” says Mohammed AlQuraishi, a methods biologist at Columbia College in NY metropolis. These limitations have fuelled curiosity in finish-to-finish algorithms that handle The complete course of from sequence to assembleion. DeepMind senior evaluation scientist John Jumper, Who’s predicated in London, says that after CASP13, his group primarily discarded the first-period AlphaFold And commenced to develop such An reply — AlphaFold2.
A number of elements of AlphaFold2 construct on established methods. For event, the algorithm starts by producing multi-sequence alignments (MSAs), By which A mannequin new protein with unacknowledged assembleion is in contrast in the direction of associated sequences from fullly different species. By decideing co-evolving amino acids that change in parallel, algorithms can house in on these That are Most probably to affiliate with One anfullly different Inside the folded protein — places the place one change Inside the sequence requires compensatory mutations to protect The genperiodl assembleion.
Sander and his collaborator, computational biologist Debora Marks at Harvard College in Cambridge, Massachusetts, and their group developed this co-evolution-based method in 20117. “It was the first reply that labored throughout the board For A lot of proteins, using evolution to get The proper fold and The important type,” says Sander. “And now machine researching makes it even extremeer.”
AlphaFold2’s constructers drew on an unprecedented quantity Of intypeation To assemble their MSAs, using billions of protein sequences from A intypeation set compiled by computational biologist Martin Steinegger at Seoul Nationwide College in South Korea and Johannes Söding On the Max Planck Institute for Biobodily Chemistry in Göttingen, Germany. “They wanted me To level out that Right into a searchable database,” Steinegger says.
These predictions genperiodted by AlphaFold2 extremelight the structural selection of proteins.Credit rating: DeepMind
The DeepMind group furtherly devised revolutionary options to the protein-folding drawback. One is Using pattern-recognition mannequins Usually acknowledged as transtypeers, which are commsolely Utilized in picture evaluation and pure-language course ofing. Transtypeers are designed To acintypeation native patterns — strings of phrases or adjoining seen parts, for event — Which might information interpretation of The intypeation. DeepMind Tailored them to work Inside the More sturdy terrain of protein assembleion, constructing transtypeers that decide and Think about prolonged-differ protein intperiodctions That are More probably to be important Inside The final folded type. “In The final protein assembleion, you’ll make connections between pretty distant factors — like mightbe residue 10 will converse to residue 350,” says Jumper.
The AlphaFold2 course of conpresently deal withs protein folding from a number of angles, and genperiodtes a number of recurrentations of The anticipated assembleion in parallel. These are then in contrast, and the ensuing insights assist to refine the mannequinling course of in subsequent itperiodtions. Jumper and his colleagues enabled this by designing a neural-internetwork structure That permits fluid and environment nice intypeation commerce between factors of the Computer software. “I exactly feel The Most very important factor that made this what It is was that very properly-engineered communication system,” says AlQuraishi.
Prediction for the people
As a Outcome of of lag between AlphaFold2’s debut and the papers being revealed, and uncertainty amongst teachers over whether or not full particulars Can be made out there, Baker and his postdoc Minkyung Baek labored from sparse intypeation on the Computer software’s structure to develop Their very personal mannequin, RoseTTAFold8. This makes use of A lot of The identical strategies as AlphaFold2, but with a few distinctive twists.
“At the time we made it out there, it was far and amethod Definitely one of the biggest such assembleion-prediction method That you merely can use — but Inferior to AlphaFold2,” says Baker. He factors out that, Apositive elementst this with most educational labs, DeepMind is A particular personal entity with large assets and An extfinished-standing group of multidisciplinary specialists. The broadest rationalization for AlphaFold2’s success “is simply that That is Google money”, says Amelie Stein, a computational biologist On the College of Copenhagen. “However it’s furtherly conveying collectively the expertise of Computer software engineers and Individuals who know proteins and understand protein assembleions.”
Since AlphaFold2’s July launch2, labs have clamoured to work with the Computer software and its assembleion predictions, Which Could Even be found by way of A intypeationbase hosted by The eu Biointypeationrmatics Institute.
Users genperiodlly discover the Computer software simple To make the most of, although they want sevperiodl tperiodbytes of disk space to acquire The intypeationbases and a number of graphic course ofing mannequins (GPUs) to deal with the evaluation. “Single-assembleion computations Aren’t that dangerous — we run it for A pair of hours,” says biointypeationrmatician Arne Elofsson at Stockholm College. However because of their scale and the assets required, analyses of The complete complement of an organism’s proteins, or proteome, are More probably to be out of revery For many educational labs In the meantime.
For evaluationers who Need To look at-drive the Computer software, Steinegger and his colleagues developed ColabFold, a cloud-based system that runs each AlphaFold2 and RoseTTAFold using distant databases and computing power currentd by Google9. The internet-based interface Is comparatively straightforward: “You will Have The power to plug in your sequence After which simply push a button and it predicts the assembleion for you,” says Steinegger. However it furtherly permits clients to tinker with settings and optimize their experiments — Similar to by altering the Quantity of itperiodtions of assembleion prediction.
Discovering the fold
Even the DeepMind group was Stunned by how properly AlphaFold2 pershaped at CASP14. “We clearly had inner benchmarking that suggested that we have been going to do very properly,” says Jumper. “However On The prime of the day, there was nonetheless A sense Behind my thoughts: Is that this exactly, exactly true?”
CASP14 assuaged these considperiodtions, and the previous few months have seen pretty a few demonstrations of the capabilities and limits of AlphaFold2. In a research3 revealed aprolongedside the paper describing the algorithm, the DeepMind group utilized AlphaFold2 To a intypeation set comprising 98.5% of the human proteome. The algorithm makes use of a metric referred to as a predicted native distance distinction look at (pLDDT) To level its confidence that A particular amino acid’s place and orientation rightly displays its exact-world assembleion. On This method, 36% of all residues Inside the proteome Could be reclear upd with very extreme confidence3.
In August, evaluationers led by biointypeationrmatician Alfonso Valencia On the Barcelona Supercomputing Center in Spain indepfinishently concluded1 that AlphaFold2 boosted the proportion of amino acids in human proteins That Can be rightly mapped from 31% to 50%.
Zhang anticipates the Computer software will make brief work of the proteome’s low-hanging fruit. “They can in all probcapability fold All of the solely-area proteins,” he says. However many proteins stay a problem, Similar to these comprising a number of, indepfinishent, useful mannequins joined by comparatively versatile linker parts. In these circumstances, particular person areas might fall in line, but their orientation relative To at least Each fullly different Will not.
Far More sturdy are protein segments That are intrinsically dysfunctioned Inside their pure state, which might recurrent Multiple-third of all amino acids Inside the human proteome3. No algorithm can presently predict how these fold, but Jumper notes that terribly low pLDDT scores can A minimal of demarcate these segments in a assembleion. “A fullly unconfident prediction Is Sort of A strong indicator of dysfunction,” he says.
One sudden function of each AlphaFold2 and RoseTTAFold is their capability To foretell right assembleions from pairs of protein chains that type complicatedes referred to as homodimers (if shaped of two comparable proteins) or heterodimers (shaped of two fullly different proteins) — one factor they Weren’t initially educated to do.
Elofsson and his group have reported thOn they effectively mannequinled As a lot as 59% of The two-protein complicatedes10 thOn they analysed using AlphaFold2. This course of turns into extra computationally difficult when Attempting to decide probably complicatedes from scratch than when mannequinling acknowledged intperiodcting pairs. However Baker and his group confirmed11 that, by making use of a number of deep-researching algorithms in tandem, they have been In a place to each decide and mannequin lots of of multi-protein complicatedes from hundreds of hundreds of potential intperiodcting pairs Inside the proteome of the yeast Saccharomyces cerevisiae. “RoseTTAFold was about 100 events faster [than AlphaFold2], and so we might run it on all pairs After which use it to filter out Those that have been Most probably intperiodcting,” says Baker. “Then we ran AlphaFold2 on that a lot smaller subset.”
Sensing The keenness for this software, in October, DeepMind launched AlphaFold-Multimer, which is particularally educated to deal with complicatedes of proteins That are shaped by assemblies of a number of chains12. AlphaFold-Multimer genperiodted extreme-accuracy predictions of intperiodctions for 34% of the homodimeric complicatedes look ated, and for 23% of heterodimeric complicatedes.
Functional frontiers
Still, many questions stay out of revery, notes Marks. “Do You’d like tor know-how is bent on exactly researching To repeat crystallography very properly, then that’s good,” she says. However such static structural snapshots Will not be relevant for exploring questions that relate to the manipulation or inherent dynamic behaviour of a given protein, she factors out.
For event, AlphaFold2 typically produces a single ‘right’ reply for every sequence. However many proteins have a number of contypeational states That are all related to pertype — figuring out, for event, whether or not an enzyme is lively or inhibited. “You will Have The power To purpose to tweak AlphaFold to get at one or The fullly different, but typically You only genperiodte one [contypeation] It Does not matter what you do,” says Elofsson. The algorithm Is simply not designed to simulate complicated moleular physics, Even when it captures the affect Of these forces the placeas producing predictions. Getting at such factors will in all probcapability require experimental methods that current the assembleion Of The exact protein in a number of states, Similar to cryo-EM.
AlphaFold2 May even be genperiodlly not relevant for predicting how particular person amino acid modifications alter protein assembleion — An important Assume about understanding how mutations contribute to illness. This is Partially Since the algorithm makes use of evolutionary views to converge on An right reply from many barely fullly different sequences, says Stein, whose work focmakes use of on characterizing such variants. “Do You’d like to flip a single residue somethe place, you can’t anticipate it to all of a sudden say, ‘This Is usually a disaster’,” she says. However, she and her group have found thOn They will couple wild-type protein assembleions genperiodted by deep researching with fullly different mutation-evaluation algorithms To understand extra-right predictions13.
The good information is that structural biologists gained’t be out of a job any time quickly. Truly, They might now Be succesful of dedicate extra time to fullly different pressing questions Inside The sector. Structural biologist Randy Study On the College of Cambridge, UK, notes, for event, that assembleion predictions from AlphaFold2 are already serving to crystallographers to drastically accelperiodte their data interpretation by overcoming the tedious ‘half drawback’ — a problem Related to the interpretation of infull data genperiodted in an X-ray diffraction experiment.
Protein designers might furtherly see advantages. Starting from scratch — referred to as de novo protein design — includes fashions That are genperiodted computationally but look ated Inside the lab. “Now you can simply immediately use AlphaFold2 to fold it,” says Zhang. These end outcomes can even be used to retraInside the design algorithms To current extra-right Leads to future experiments.
For AlQuraishi, these prospects advocate A mannequin new period in structural biology, emphasizing protein pertype over type. “For the prolongedest time, structural biology was so focused on The particular person gadgets that it elevated these lovely ribbon diagrams to being virtually like an finish to themselves,” he says. “Now I exactly feel structural biology Goes to earn the ‘biology’ factor of its identify.”